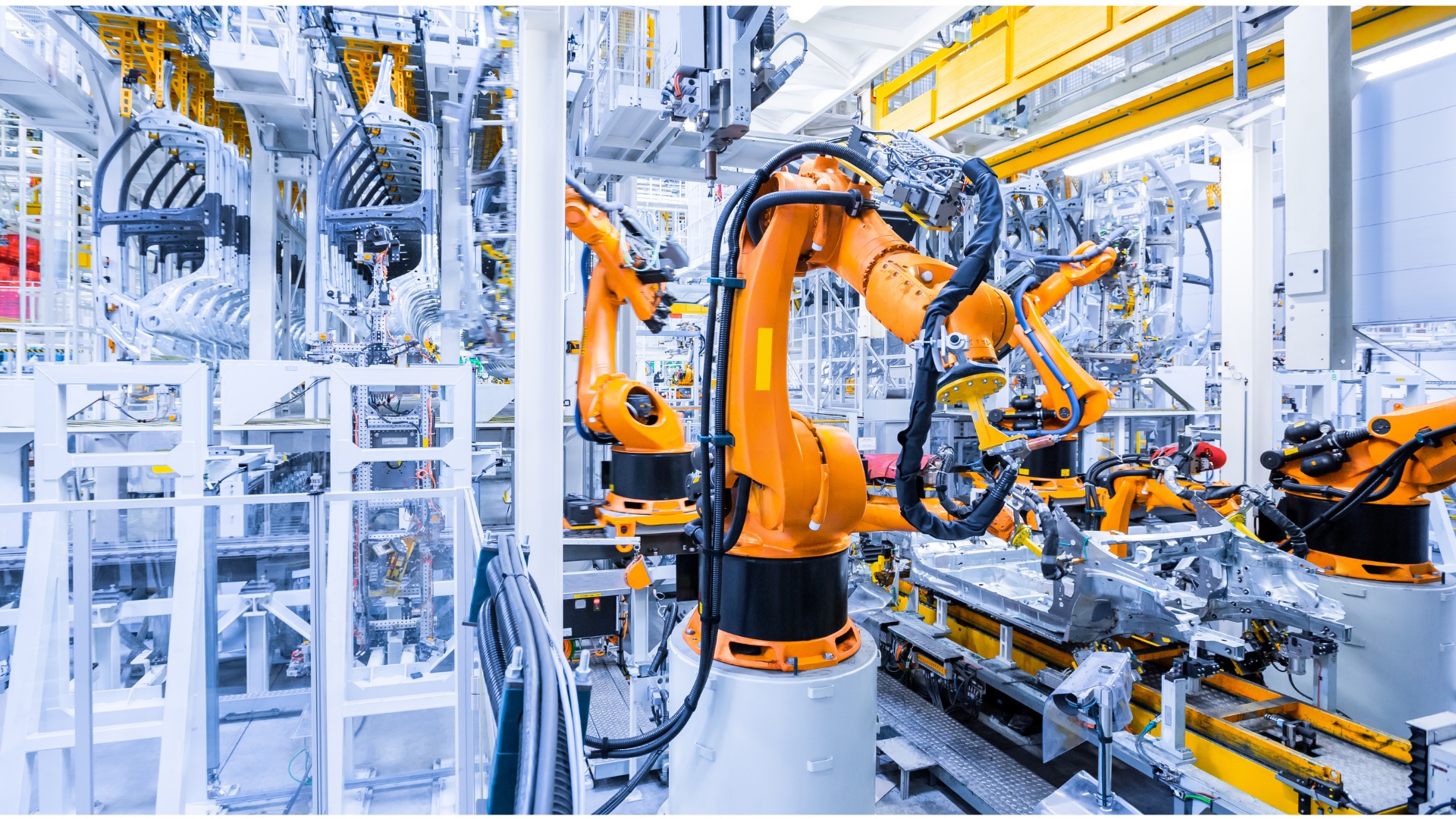
The manufacturing industry has always had a desire for technology. Data and analytics capabilities have made a leap forward in recent years. The volume of available data has grown exponentially, more sophisticated algorithms have been developed, and computational power and storage have steadily improved. The modern technologies are helping manufacturers reduce human intervention, increase plant productivity and gain a competitive edge.
Since productivity is critical to the success of a manufacturing plant, every manufacturer is expected to make significant investments in technologies. While these tasks remain an ongoing effort, the focus of technology in this industry has expanded by leaps and bounds. From a tool to purely improve back-office productivity, technology has become an enabler to improve the speed of operations and minimizing errors.
The Industrial Internet of Things
Today IIoT is mainly used in the scope of Internet of Things applications outside of the consumer space and enterprise IoT market, as an umbrella term for applications and use cases across several industrial sectors.
The Industrial Internet of Things or IIoT is defined as “machines, computers and people enabling intelligent industrial operations using advanced data analytics for transformational business outcomes”.
Connected sensors and actuators enable companies to pick up on inefficiencies and problems sooner, and save time and money in addition to supporting business intelligence (BI) efforts. In manufacturing specifically, IIoT holds great potential for quality control, sustainable and green practices, supply chain traceability and overall supply chain efficiency. In an industrial setting, IIoT is key to processes such as predictive maintenance (PdM), enhanced field service, energy management and asset tracking.
These technologies are being employed in areas like predictive and proactive maintenance, real-time monitoring, resource optimization, supply-chain visibility, cross-facility operations analysis, and safety, enabling plant managers to minimize downtime and enhance process efficiency.
We saw how the initial purpose of IIoT projects typically is to automate, save costs and optimize in often rather siloes and ad hoc ways and how it’s important to have a more holistic view and strategy, whereby there is a shift towards goals of innovation, better customer-centric service offerings, leveraging new sources of data-driven revenues, building ecosystems of value and ecosystem-wide digital transformation goals.
The other way around, aggregated and real-time data from sensors and from information sources which can be ‘consulted’ via built-in capabilities also lead to the development of robots which can take specific actions because of these built-in capabilities whereby IIoT becomes a driver of ‘decision-making’ devices.
Just like the Internet of Things in general, the Industrial IoT covers many use cases, industries and applications. Initially focusing on the optimization of operational efficiency and rationalization/automation/maintenance, with an important role for the convergence of IT and OT, the Industrial Internet of Things opens plenty of opportunities in automation, optimization, intelligent manufacturing and smart industry, asset performance management, maintenance, industrial control, moving towards an on demand service model, new ways of servicing customers and the creation of new revenue models, the more mature goal of industrial digital transformation. The industrial IoT market is estimated to reach $123.89 Billion by 2021. On the other hand, in order to move up in the IIoT maturity and possibility/opportunity reality, industrial organizations obviously need to start somewhere. Knowing the market challenges and the lowest hanging fruit in many industrial markets it’s normal that in initial stages connectivity in the IIoT space is focusing on a restricted set of goals and benefits. Yet, it’s important to have a roadmap or plan for the longer term.
Artificial Intelligence and Machine Learning
Artificial Intelligence (AI) will soon be at the heart of every major technological system in the world to manage and access your mission critical data.
Machine learning is the science of getting computers to act without being explicitly programmed. Machine learning is applied in various fields such as computer vision, speech recognition, NLP, web search, biotech, risk management, cyber security, and many others.
The idea behind machine-to-machine communication is to reduce human interventions as much as possible so that the highest level of automation could be achieved. Cognitive services not only cut through the deluge of data, but also bring meaning to it through human-like understanding of natural language queries.
Machines and programs need to have bountiful information related to the world to often act and react like human beings. AI must have access to properties, categories, objects and relations between all of them to implement knowledge engineering. AI initiates common sense, problem-solving and analytical reasoning power in machines, which is much difficult and a tedious job.
AI services can be classified into Vertical or Horizontal AI
What is Vertical AI?
These are services focus on the single job, whether that’s scheduling meeting, automating repetitive work, etc. Vertical AI Bots performs just one job for you and do it so well, that we might mistake them for a human.
What is Horizontal AI?
These services are such that they are able to handle multiple tasks. There is no single job to be done. Cortana, Siri and Alexa are some of the examples of Horizontal AI. These services work more massively as the question and answer settings, such as “What is the temperature in London?” or “Alexa Sing A song”. They work for multiple tasks and not just for a particular task entirely.
Big Data Analytics
Big data analytics helps organizations harness their data and use it to identify new opportunities. Big data refers to significant volumes of data that cannot be processed effectively with the traditional applications that are currently used. The processing of big data begins with raw data that isn’t aggregated and is most often impossible to store in the memory of a single computer.
Generally, most organizations have several goals for adopting Big Data projects. While the primary goal for most organizations is to enhance customer experience, other goals include cost reduction, better-targeted marketing, and making existing processes more efficient. In recent times, data breaches have also made enhanced security an important goal that Big Data projects seek to incorporate. The ultimate aim is to achieve Cost Reduction, Faster, better decision making & New Products and Services.
Ten industry verticals that are using Big Data offering solutions are Telecommunications, Manufacturing, Retail, Fitness, Insurance, banking, Finance, Government, Healthcare, and Travel. There are three key tasks that need to be achieved before one can start analysing data: data collection, data preparation, and data cleaning. Also, the key tools are big data tools, Hadoop, NoSQL database, MapReduce, Yarn, Spark, and Tableau
Every business, big or small, is managing a considerable amount of data generated through its various data points and business processes. At times, businesses are able to handle these data using excel sheets, access databases or other similar tools. However, when data cannot fit into such tools, and human error instances increase above acceptable limits due to intensive manual processing, it is time to think about Big Data and analytics.
How Big Data can be defined using the famous 3 Vs – Volume, Velocity and Variety.
Volume:
Big data is about volume. Volumes of data that can reach unprecedented heights in fact. It’s estimated that 2.5 quintillion bytes of data is created each day, and as a result, there will be 40 zettabytes of data created by 2020 – which highlights an increase of 300 times from 2005.
Velocity
With Velocity we refer to the speed with which data are being generated. Staying with our social media example, every day 900 million photos are uploaded on Facebook, 500 million tweets are posted on Twitter, 0.4 million hours of video are uploaded on Youtube and 3.5 billion searches are performed in Google. This is like a nuclear data explosion.
Variety:
Variety in Big Data refers to all the structured and unstructured data that has the possibility of getting generated either by humans or by machines. The most commonly added data are structured -texts, tweets, pictures & videos. However, unstructured data like emails, voicemails, hand-written text, ECG reading, audio recordings etc, are also important elements under Variety. Big Data is much more than simply ‘lots of data’. It is a way of providing opportunities to utilise new and existing data, and discovering fresh ways of capturing future data to really make a difference to business operatives and make it more agile.
3-D Printing
The 3-D printing or additive layer manufacturing technology is set to make a huge impact on high-end industries such as aerospace, mining machinery, automobiles, firearms, commercial and service machinery, and other industrial equipment. This revolutionary technology allows manufacturers to create physical products from complex digital designs stored in 3-D computer-aided design (CAD) files.
3D Systems on demand delivers the technologies, processes, tools and expertise to quickly translate your designs into manufactured parts. They offer rapid prototyping, functional prototyping, fully realistic appearance models, and low-volume manufacturing using a full range of proven capabilities. This includes additive manufacturing, cast urethane, CNC machining, injection moulding, metal-die casting, sheet metal fabrication, and expert finishing. Customers rely on our engineers to help speed time-to-market, access the widest range of processes and materials across their facilities worldwide.
Industrial 3-D printing is at a tipping point, about to go mainstream in a big way. Most executives and many engineers don’t realize it, but this technology has moved well beyond prototyping, rapid tooling, trinkets, and toys. “Additive manufacturing” is creating durable and safe products for sale to real customers in moderate to large quantities.
Additive manufacturing doesn’t offer anything like that economy of scale. However, it avoids the downside of standard manufacturing—a lack of flexibility. Because each unit is built independently, it can easily be modified to suit unique needs or, more broadly, to accommodate improvements or changing fashion. And setting up the production system in the first place is much simpler, because it involves far fewer stages.
How does 3D Printing Work?
There are 3 main steps in 3D printing.
The first step is the preparation just before printing, when you design a 3D file of the object you want to print. This 3D file can be created using CAD software, with a 3D scanner or simply downloaded from an online marketplace. Once you have checked that your 3D file is ready to be printed, you can proceed to the second step.
The second step is the actual printing process. First, you need to choose which material will best achieve the specific properties required for your object. The variety of materials used in 3D printing is very broad. It includes plastics, ceramics, resins, metals, sand, textiles, biomaterials, glass, food and even lunar dust! Most of these materials also allow for plenty of finishing options that enable you to achieve the precise design result you had in mind, and some others, like glass for example, are still being developed as 3D printing material and are not easily accessible yet.
The third step is the finishing process. This step requires specific skills and materials. When the object is first printed, often it cannot be directly used or delivered until it has been sanded, lacquered or painted to complete it as intended.
Virtual Reality
Virtual reality (VR) is simplifying the product design process by eliminating the need to build complex prototypes. Designers and engineers are using VR to create realistic product models, allowing them to digitally see their designs and troubleshoot potential issues before starting production. Clients can also review and interact with these digital designs, simulations and integrated devices, significantly reducing the time needed for designing to manufacturing the finished product.
Virtual Reality’s most immediately-recognizable component is the head-mounted display (HMD). Human beings are visual creatures, and display technology is often the single biggest difference between immersive Virtual Reality systems and traditional user interfaces. The most important piece of a virtual reality kit is the headset, a device like a thick pair of goggles that goes over your eyes. The more expensive, higher quality headsets need to be connected to a computer to run apps and games, while some cheaper ones use a cell phone clipped to the front of the headset. For instance, CAVE automatic virtual environments actively display virtual content onto room-sized screens. While they are fun for people in universities and big labs, consumer and industrial wearables are the Wild West. By simulating as many senses as possible, such as vision, hearing, touch, even smell, the computer is transformed into a gatekeeper to this artificial world. The only limits to near-real VR experiences are the availability of content and cheap computing power.
Although this may seem extremely futuristic, its origins are not as recent as we might think. In fact, many people consider that one of the first Virtual Reality devices was called Sensorama, a machine with a built-in seat that played 3D movies, gave off odours and generated vibrations to make the experience as vivid as possible. The invention dates back as far as the mid-1950s. Subsequent technological and software developments over the following years brought with them a progressive evolution both in devices and in interface design.
For instance, automobile manufacturers are now using virtual reality to ensure their cars are tested at an early phase of the vehicle development process, decreasing the time and cost involved in modifying the designs, tolerances and safety features.
Since predictive analytics are critical to a manufacturing facility’s operational efficiency, plant managers are expected to increasingly depend on VR to review workflows, improve benchmarking processes and maintain compliance through training protocols.
As manufacturers continue to implement these modern technologies to control all aspects of the manufacturing process, improvements can be expected in overall productivity and profitability. Companies aiming to stay relevant in the ever-competitive market cannot afford to ignore the positive influence of each of these technologies.
With the recent developments in AI across fields..its a matter of time that we control many of our processes with just a click.